What if the cure is a drug that already exists?
Using advanced technology and data, researchers are repurposing drugs for rare diseases, cancer and more
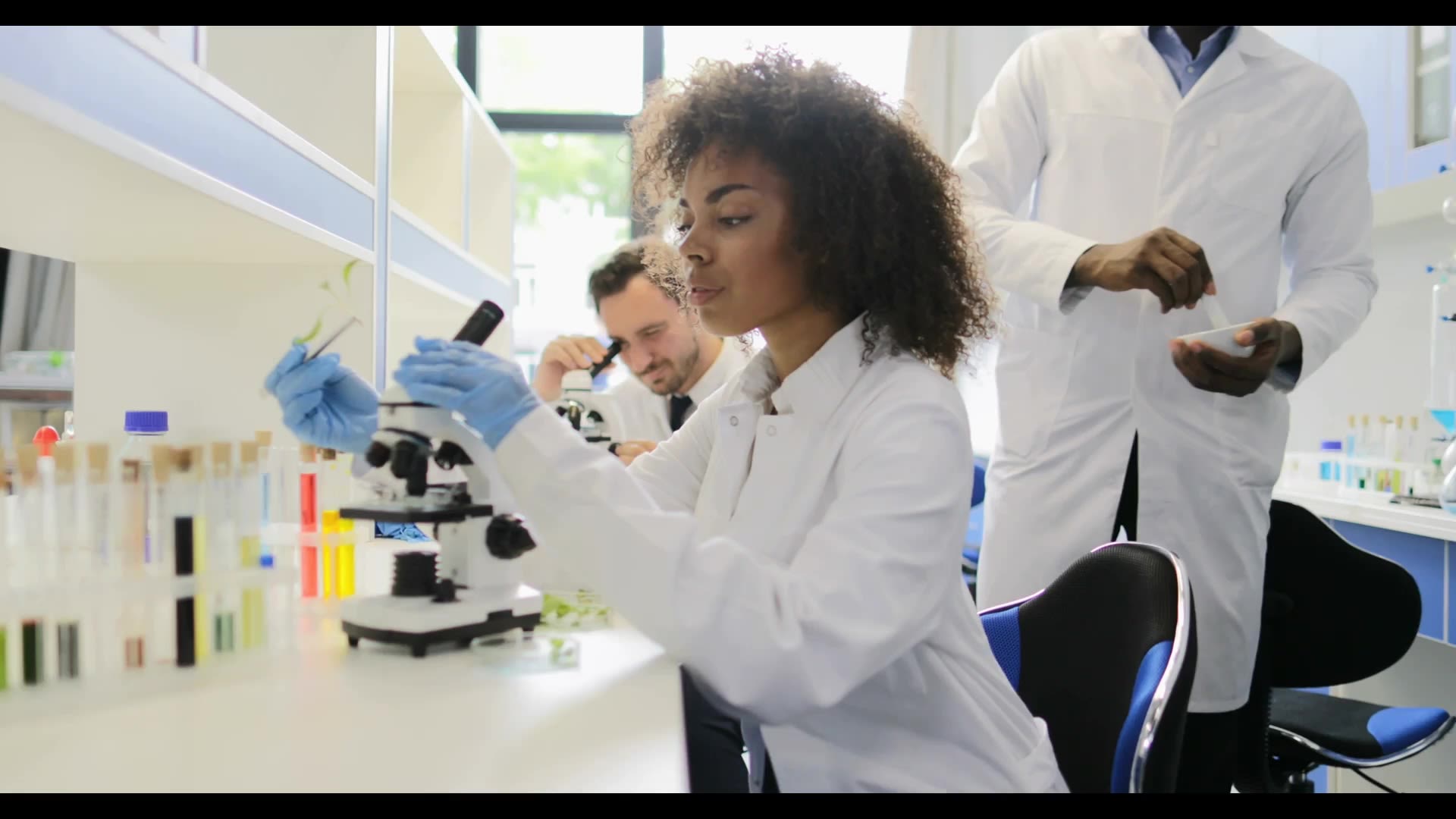
The search for cures has never been more pressing. With over 9,000 rare diseases afflicting millions of people worldwide, the toll on patients and their families immeasurable. Add to that the growing burden of cancer: About one in five people develop cancer in their lifetime, but the majority of countries do not cover basic cancer care as part of health services for all citizens, according to the World Health Organization.
To find cures more quickly and reduce the cost, some researchers are turning to drug repurposing — an innovative approach that offers new hope for those who suffer from diseases once thought untreatable. While repurposing has a long and rich history, Elsevier is now applying AI and data science to reinvent and accelerate the process, providing data and algorithms to innovative partners in industry and the nonprofit sector. Here’s a glimpse of what we’re accomplishing together.
Why rare diseases are posing a massive societal challenge
By Jen Tidman | eUROGEN
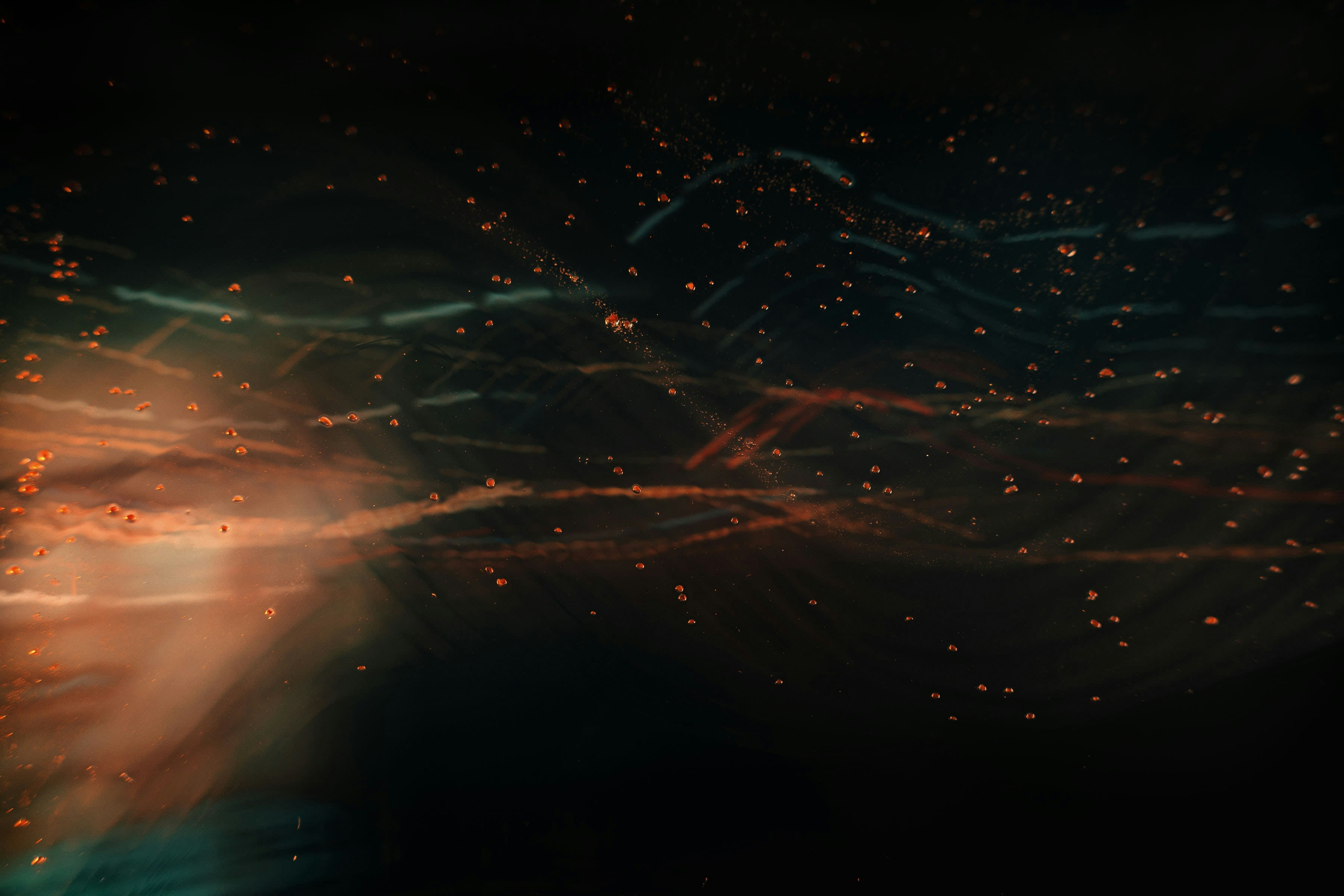
In the United States, a disease is considered rare if it affects fewer than 200,000 people at any given time, and in the European Union, if it affects fewer than one in 2,000 people. While each rare disease affects a relatively low number of people, however, they have a huge collective impact, with an estimated 36 million individuals in the EU alone grappling with rare diseases.
Also known as orphan diseases, they affect individuals across age groups, races and genders. They can stem from genetic, environmental, infectious or unknown origins, presenting chronic, progressive and life-threatening challenges. Rare diseases present numerous societal challenges, including limited access to care, diagnostic delays, treatment issues, financial burden, and lack of education and awareness.
The European Reference Networks (ERNs) are virtual networks connecting healthcare providers, researchers and patients across borders to improve diagnoses and treatment of rare diseases and complex conditions.
The European Reference Networks (ERNs) are virtual networks connecting healthcare providers, researchers and patients across borders to improve diagnoses and treatment of rare diseases and complex conditions.
Addressing these societal challenges requires a multifaceted approach involving stakeholders across the healthcare system, government agencies, research institutions, industry, and patient advocacy organizations. Efforts to raise awareness, improve access to care, increase research funding and support innovation are critical for addressing the needs of individuals and families affected by rare diseases.
“Rare diseases present numerous societal challenges largely due to their complex nature, low prevalence and often devastating impacts on individuals and families.”
A doctor finds a cure for his own rare disease — and now others
By Ann-Marie Roche
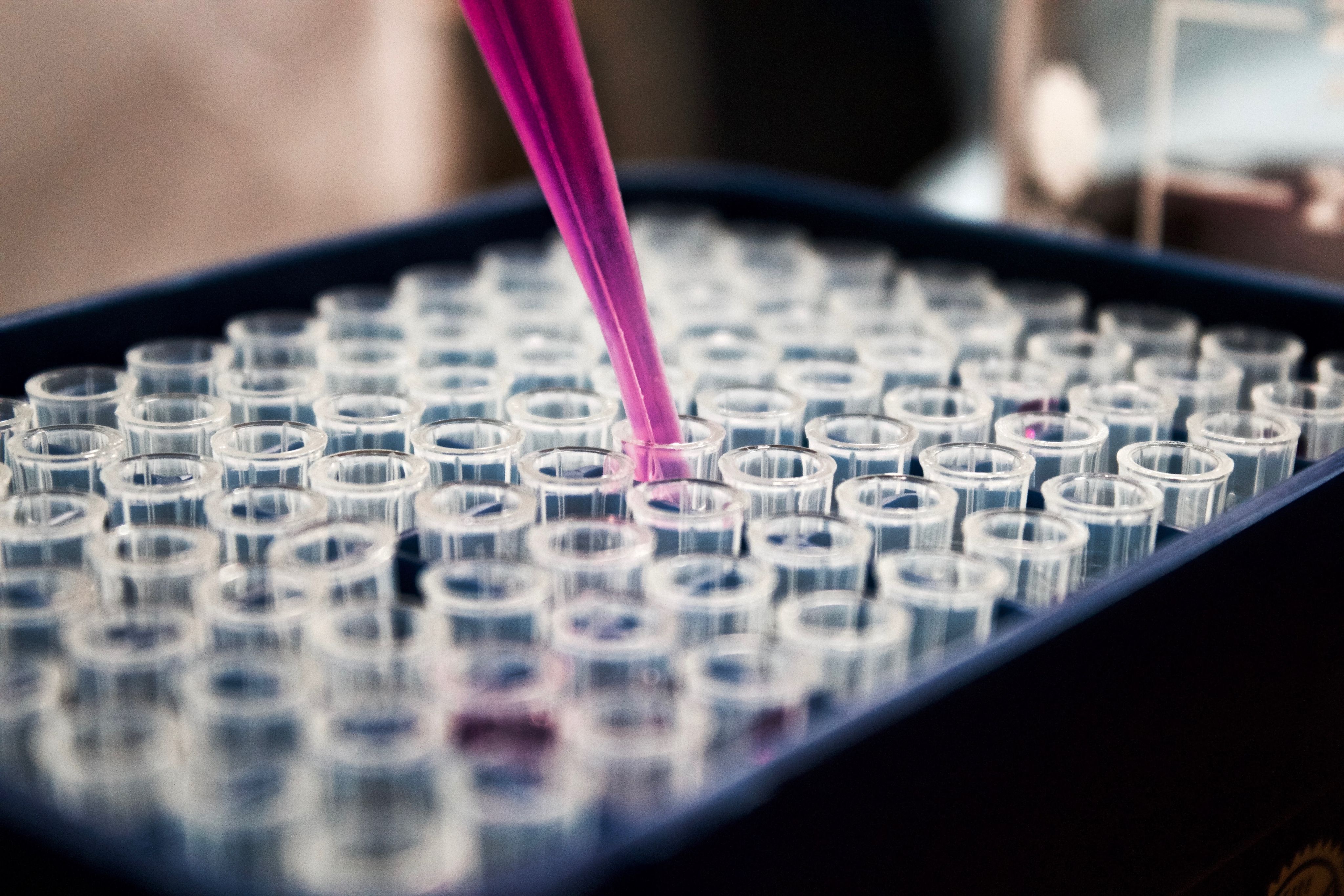
“It’s an incredible opportunity that’s been hiding in plain sight. Now it’s time for as many of us as possible to help make it happen as soon as possible.”
Dr David Fajgenbaum tells his story at the 2023 STAT Breakthrough Summit. Watch the video here
Dr David Fajgenbaum saved his own life by tracking down a generic drug that cured his rare and deadly disease. Now with nonprofit Every Cure — and Elsevier’s data and algorithms — he’s seeking cures for the estimated 9,000+ untreatable rare diseases.
“These are some of the patients that are alive today because of drugs that we repurposed for them.” — Every Cure Founder David Fajgenbaum, MD
“These are some of the patients that are alive today because of drugs that we repurposed for them.” — Every Cure Founder David Fajgenbaum, MD
It’s a riveting read: Chasing My Cure: A Doctor’s Race To Turn Hope Into Action is Dr David Fajgenbaum’s memoir about almost dying five times from idiopathic multicentric Castleman disease (iMCD) before discovering his own treatment in the form of a disused drug.
His continued remission — 10 years and counting — means he can continue to focus on Every Cure, a nonprofit he co-founded. He and his team are developing an AI-driven tool using a variety of algorithms to scour all relevant knowledge databases to look for potential matches between each of the 3,000+ FDA-approved drugs and a specific incurable disease of the 9,000+ currently documented. Each match is given a predictive efficacy score. The highest-scoring pairs will then be studied to see if they warrant more research and potentially clinical trials.
Elsevier is providing data and its algorithms to the project. Initially, Every Cure provided a list of 10 incurable diseases — ranging from iMCD to sepsis to autism. Elsevier’s Professional Services Group customized algorithms to follow a disease path for what drugs could be repurposed for what diseases. And here’s a fun fact: the algorithms tagged the same drug in seconds that David struggled to find manually to treat his iMCD.
Repurposing drugs for rare diseases, cancer and beyond
By Ann-Marie Roche
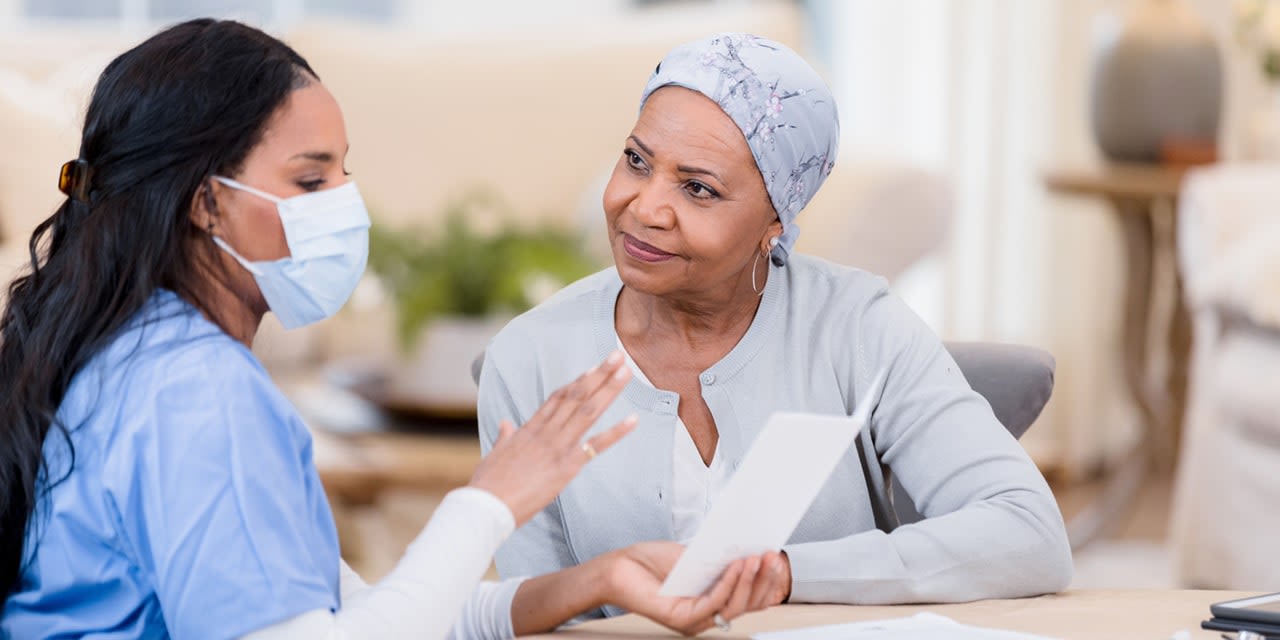
Elsevier is applying AI and data science to reinvent and accelerate the process of drug repurposing.
It’s an enticing concept: recycling old drugs for new cures. It’s also viable: aspirin, Viagra and the new weight loss drug Wegovy are all repurposed drugs. And today, thanks to innovations in AI, we are accelerating the process — call it digital speed-dating that links a disease with its potential treatment.
But what does using precision medicine for crop protection have to do with it? We talked to colleagues on Elsevier’s Professional Services team to find out.
“Swiss Army” medicine
When we asked Elsevier’s VP of Data Science Mark Sheehan what non-AI related innovation is exciting him the most lately, his eyes lit up:
“Novo Nordisk’s weight loss drug Wegovy. It’s amazing how it’s tapped into a massive new market — a rarity in the life sciences. You have to be impressed, especially when it’s answering a specific global crisis: obesity.”
Wegovy is also interesting because it began as a treatment for diabetes. Now it’s been repurposed — like aspirin, Viagra, thalidomide and countless other drugs before it. It’s also interesting because drug repurposing — aka drug repositioning or reprofiling — can help meet the global demand for unmet medical needs.
By reducing timelines and costs compared to creating drugs from scratch, repurposing also taps into a deeply human emotion: the love for a bargain. Why spend millions on R&D if a solution already exists?
And while repurposing enjoys a long and rich history, Elsevier is now applying AI and data science to reinvent and accelerate the process, with the Professional Services team at the forefront of this quest. In fact, the related work has expanded beyond healthcare to also take in agriculture and confronting climate change.
“It’s a passion we all have here in Professional Services. It comes down to Elsevier having a huge mass of data, and many different tools to access this data. And my primary job is actually to help customers navigate this maze of products, databases, interfaces, and so on — taking this immense amount of data and knowledge, focusing it, and then applying it to cure patients.”
How knowledge graphs can supercharge drug repurposing
By Joe Mullen, PhD
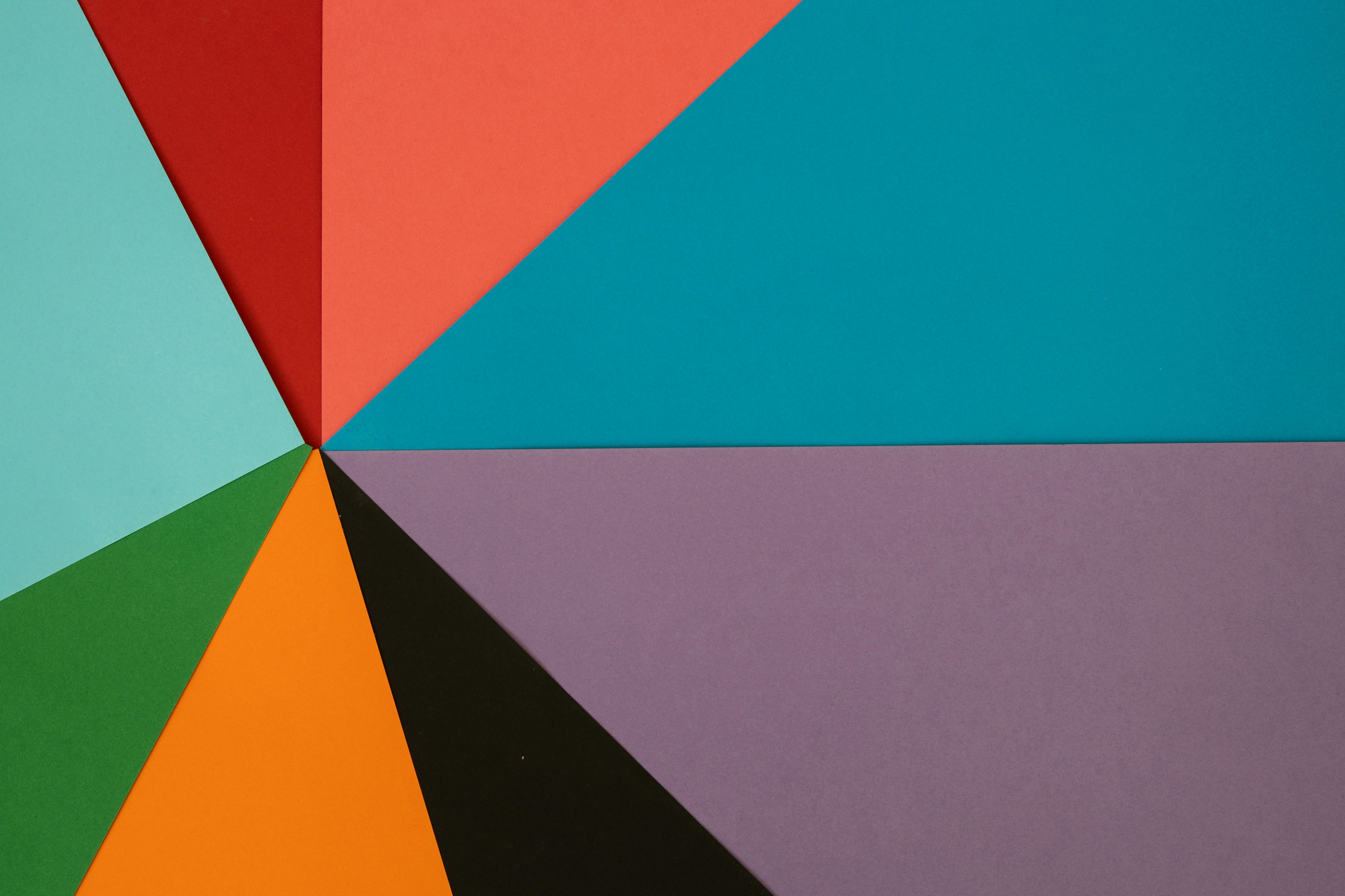
“Knowledge graphs are a powerful data science technology that connects data to visually represent a network of entities and relationships. Importantly, knowledge graphs capture facts. This is vital for evidence-based decisions in fields like life sciences, where bad decisions can have dire consequences.
5 questions to ask
To use knowledge graphs successfully in drug repurposing, you need to address these questions:
- Is our organization ready? Have we identified the specific question we are looking to answer or is the knowledge graph a hammer looking for a nail?
- What data do we have and what data do we need? Have we considered a breadth of sources, or do we need to source more data internally and externally to answer the research question?
- How will the knowledge graph be used? Do we want a list of answers, or do we want the knowledge graph? And what UI will be required if internal users are given access to it? This ensures the outputs are used in the way you intend by the people you intend.
- Have we laid the groundwork of foundational data technologies? This is a crucial step. Are data mapped to ontologies, semantically enriched, and aligned with public IDs?
- What skills do we have and what help do we need? A successful knowledge graph and underlying foundational technologies are typically beyond the in-house data science skills of many pharmaceutical companies. Organizations will often bring in external expertise to build effective knowledge graphs.
Knowledge graphs offer multidirectional insight into drugs, diseases and targets to accelerate drug repurposing — but organizations must address key questions to benefit from this technology.
This Sankey diagram was produced from data within Elsevier’s Biology Knowledge Graph and shows relationships among the disease endometrial cancer and associated entities, including drugs and proteins. (Source: EmBiology)
This Sankey diagram was produced from data within Elsevier’s Biology Knowledge Graph and shows relationships among the disease endometrial cancer and associated entities, including drugs and proteins. (Source: EmBiology)
Finding new uses for existing drugs reduces the time and cost of developing new therapies and benefits patients by bringing them to market sooner. But drug repurposing is more complex than it sounds, and many companies struggle to bring projects to fruition. Often, this is because they lack a data and search strategy that enables deep, multidirectional insight into what we call the drug repurposing triumvirate: drug, disease, target.
It is essential that researchers can visualize and analyze the complex relationships between these entities. Doing so requires a search strategy that goes beyond a 2D “flat” search via a traditional relational database. It demands a purpose-built solution that can handle domain specific terminology. The answer — knowledge graphs: the “3D” search upgrade for drug repurposing.
The intuitive power of a knowledge graph
Knowledge graphs are a powerful data science technology that connects data to visually represent a network of entities and relationships. Importantly, knowledge graphs capture facts. This is vital for evidence-based decisions in fields like life sciences, where bad decisions can have dire consequences. This also differentiates knowledge graphs from other approaches such as open-source large language models (LLMs), where veracity is not guaranteed.
However, there can be considerable interplay between knowledge graphs and private LLMs to the benefit of researchers. LLMs can aid in the generation of a knowledge graph and can lower the barrier to entry when it comes to interrogation of graphs, enabling users of all experience levels to benefit from knowledge graphs. For example, converting natural language prompts into native graph query languages, such as Cypher, enables non-data science experts to question a knowledge graph.
In a repurposing scenario, a knowledge graph is an intuitive and, importantly, explainable way of viewing the drug, disease, target triumvirate. Building a knowledge graph requires foundational data technologies to be in place. The first step is mapping equivalent concepts from different data sources into ontologies. Ontologies are a technique for organizing data beyond lists and thesauri to categorize concepts based on a logical, shared framework. This logic-based way of defining concepts produces a shared vocabulary that is machine processable.
The next step is the application of semantic annotation tools to scan vast quantities of scientific publications and then normalize scientific concepts to unique entity IDs. These IDs are mapped to public standards, so all evidence mined from the literature, alongside supporting evidence from the public domain and internal data systems, can be pulled together to be presented in a searchable knowledge graph.
The logic-based collation and organization of data in a knowledge graph allows researchers to look at drug repurposing questions “in the round,” from every angle. The benefits of this 3D approach are:
- More relevant search results: Data organized in a knowledge graph powers more accurate and relevant answers to user queries.
- Easier data integration: Data connected from multiple sources, both internal and external, are integrated in a single destination via a unified view.
- Enables discovery: The ability to infer relationships and connections can reveal new insights that weren’t visible before. While flat search of a relational database only shows the relationship between A–B, and B–C, a knowledge graph’s underpinning logic also infers the hidden relationship between A–C.
- Powers AI and machine learning: Knowledge graphs support further adoption of advanced technology including AI/ML, natural language queries, automation and scenario modelling.
- Exposes gaps or errors: It’s easier to visually identify gaps or data errors with a knowledge graph, which can be subsequently amended to improve data quality and accuracy.
- Traces data provenance: The user can see where every relationship has come from, enabling evidence-based decision making.
- Dynamically updated: Data pipelines that feed knowledge graphs are updated automatically and in real-time, so researchers are sure decisions are made based on the latest scientific data.